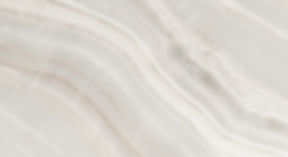
Abstract
Image-text matching is a fundamental and crucial problem in multi-modal information retrieval. Although much progress has been made in bridging vision and language, it remains challenging because of the requirements for the intra-modal reasoning and cross-modal alignment. Despite different modality interaction patterns have been explored, there are still many effective interaction patterns that have not been considered. Besides, existing methods depend heavily on expert experience towards the design of interaction patterns, therefore lacking flexibility.
To address these issues, we develop a novel modality interaction modeling network relying on the dynamic routing technology, which is the first unified and dynamic multimodal interaction framework for image-text matching. In particular, we first design four types of cells to explore different levels of modality interactions, and then connect them in a dense way to construct a routing space. To endow the model with path decision capability, we integrate a dynamic router in each cell for pattern exploration. As the routers are conditioned on inputs, our model can dynamically learn different activated paths for different data. Extensive experiments on two benchmark datasets, i.e., Flickr30K and MS-COCO, demonstrate the effectiveness and superiority of our model compared with the state-of-the-art methods.

Framework
-
Codes
DIME
-
Datasets
Flickr30K
-
Pretrained Models